$2 H100s: How the GPU Rental Bubble Burst
Last year, H100s were $8/hr if you could get them. Today, there's 7 different resale markets selling them under $2. What happened?
Don’t miss Eugene’s responses on the HN and Reddit and Twitter discussions!
Swyx’s note: we’re on a roll catching up with former guests! Apart from our recent guest spot on Raza Habib’s chat with Hamel Husain (see our Raza pod here), we’re delighted for the return of Eugene Cheah (see our pod on RWKV last year) as a rare guest writer for our newsletter. Eugene has now cofounded Featherless.AI, an inference platform with the world’s largest collection of open source models (~2,000) instantly accessible via a single API for a flat rate (starting at $10 a month).
Recently there has been a lot of excitement with NVIDIA’s new Blackwell series rolling out to OpenAI, with the company saying it is sold out for the next year and Jensen noting that it could be the “most successful product in the history of the industry”. With cousin Lisa hot on his heels announcing the MI3 25 X and Cerebras filing for IPO, it is time to dive deep on the GPU market again (see also former guest Dylan Patel’s pod for his trademark candid take of course - especially his take on what he calls the “AI Neoclouds”, which is what Eugene discusses here).
Do we yet have an answer to the $600bn question? It is now consensus that the capex on foundation model training is the “fastest depreciating asset in history”, but the jury on GPU infra spend is still out and the GPU Rich Wars are raging. Meanwhile, we know now that frontier labs are spending more on training+inference than they make in revenue, raising $6.6b in the largest venture round of all time while also projecting losses of $14b in 2026. The financial logic requires AGI to parse.
Fortunately, we’ve got someone who spends all his time thinking about this. What follows is Eugene’s take on GPU economics as he is now an inference provider, diving deep on the H100 market, as a possible read for what is to come for the Blackwell generation. Not financial advice! We also recommend Yangqing Jia’s guide.
TLDR: Don’t buy H100s. The market has flipped from shortage ($8/hr) to oversupplied ($2/hr), because of reserved compute resales, open model finetuning, and decline in new foundation model co’s. Rent instead1.
For the general market, it makes little sense to be investing in new H100s today, when you can rent it at near cost, when you need it, with the current oversupply.
A short history of the AI race
ChatGPT was launched in November 2022, built on the A100 series. The H100s arrived in March 2023. The pitch to investors and founders was simple: Compared to A100s, the new H100s were 3x more powerful, but only 2x the sticker price.
If you were faster to ramp up on H100s, you too, can build a bigger, better model, and maybe even leapfrog OpenAI to Artificial General Intelligence - If you have the capital to match their wallet!
With this desire, $10-100’s billions of dollars were invested into GPU-rich AI startups to build this next revolution. Which lead to ….
The sudden surge in H100 demand
Market prices shot through the roof, the original rental rates of H100 started at approximately $4.70 an hour but were going for over $8. For all the desperate founders rushing to train their models to convince their investors for their next $100 million round.
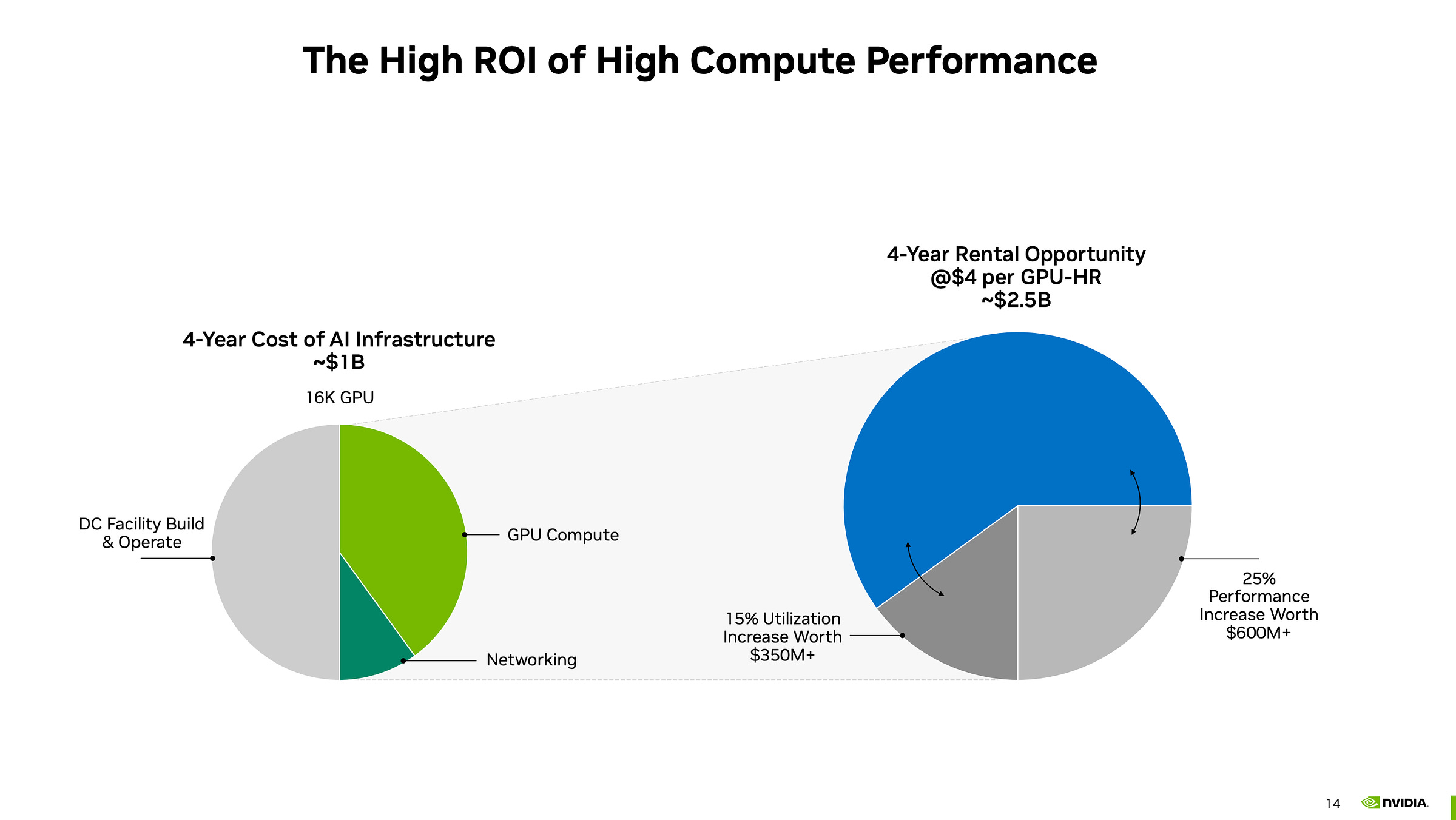
For GPU farms, it felt like free money - if you can get these founders to rent your H100 SXMGPUs at $4.70 an hour or more, or even get them to pay it upfront, the payback period was <1.5 years. From then on, it was free-flowing cash of over $100k per GPU, per year.
With no end to the GPU demand in sight, their investors agreed, with even larger investments2…
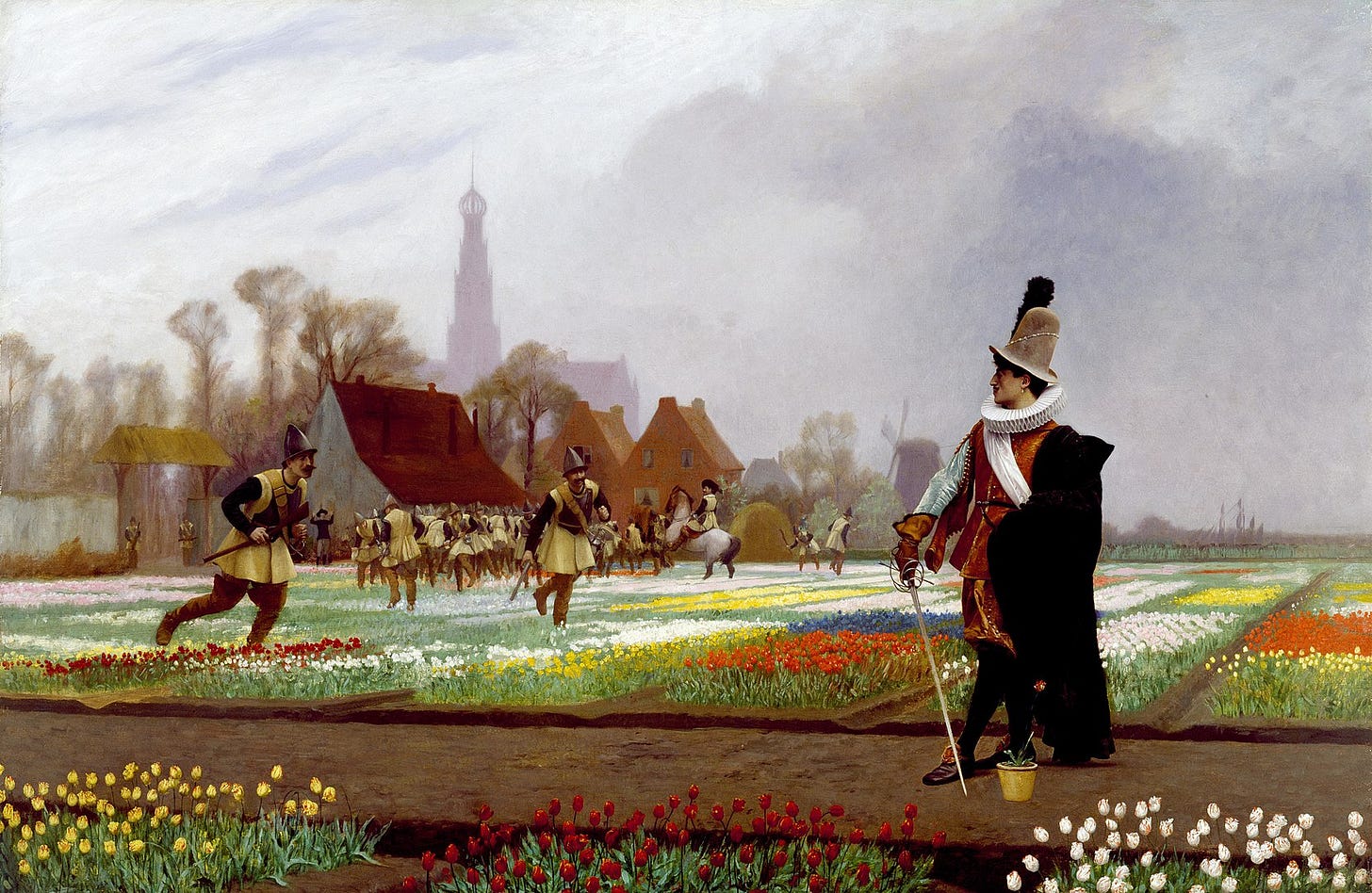
$600 Billion in investment later …
Physical goods, unlike digital goods, suffer from lag time. Especially when there are multiple shipment delays.
For most of 2023, the H100 prices felt like they would forever be above $4.70 (unless you were willing to do a huge upfront downpayment)
At the start of 2024, the H100 prices reached approximately $2.85 across multiple providers.
As more providers come online, however… I started to get emails like this:
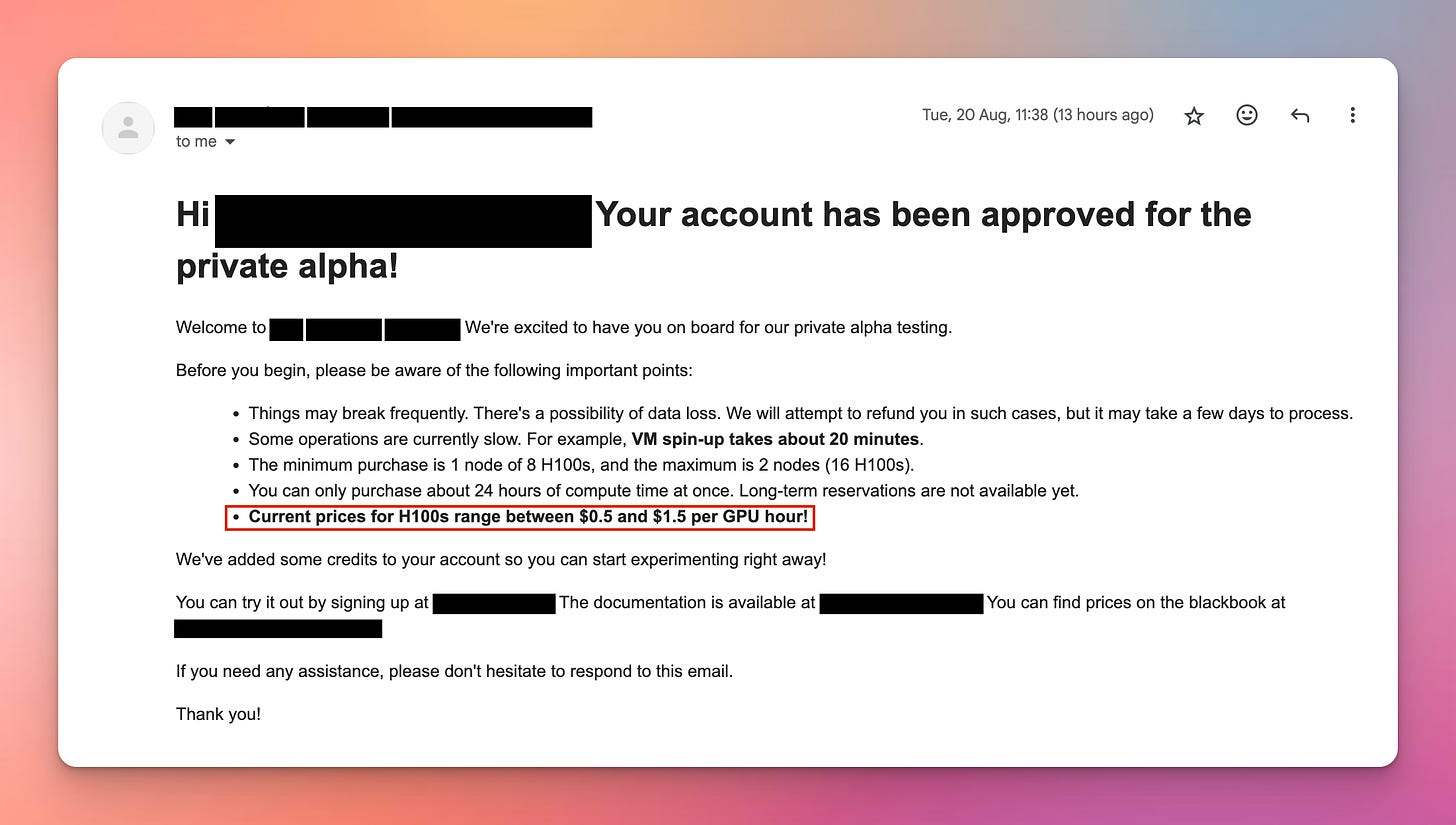
In Aug 2024, if you're willing to auction for a small slice of H100 time (days to weeks), you can start finding H100 GPUs for $1 to $2 an hour.
We are looking at a >= 40% price drop per year, especially for small clusters. NVIDIA’s marketing projection of $4 per GPU hour across 4 years, has evaporated away in under 1.5 years.
And that is horrifying because it means someone out there is potentially left holding the bag - especially so if they just bought it as a new GPUs. So what is going on?
What’s the ROI on a USD $50k H100 SXM GPU?
This will be focusing on the economical cost, and the ROI on leasing, against various market rates. Not the opportunity cost, or buisness value.
The average H100 SXM GPU in a data center costs $50k or more to set up, maintain, and operate (aka most of the CAPEX). Excluding electricity and cooling OPEX cost. More details on the calculation are provided later in this article.
But what does that mean for unit economics today, as an investment?
Especially if we assume a 5-year lifespan on the GPUs itself today.
Generally, there are two business models for leasing H100, which we would cover.
Short on-demand leases (by the hour - by the week - or the month)
Longterm reservation (3-5 years)
On-demand leasing ROI
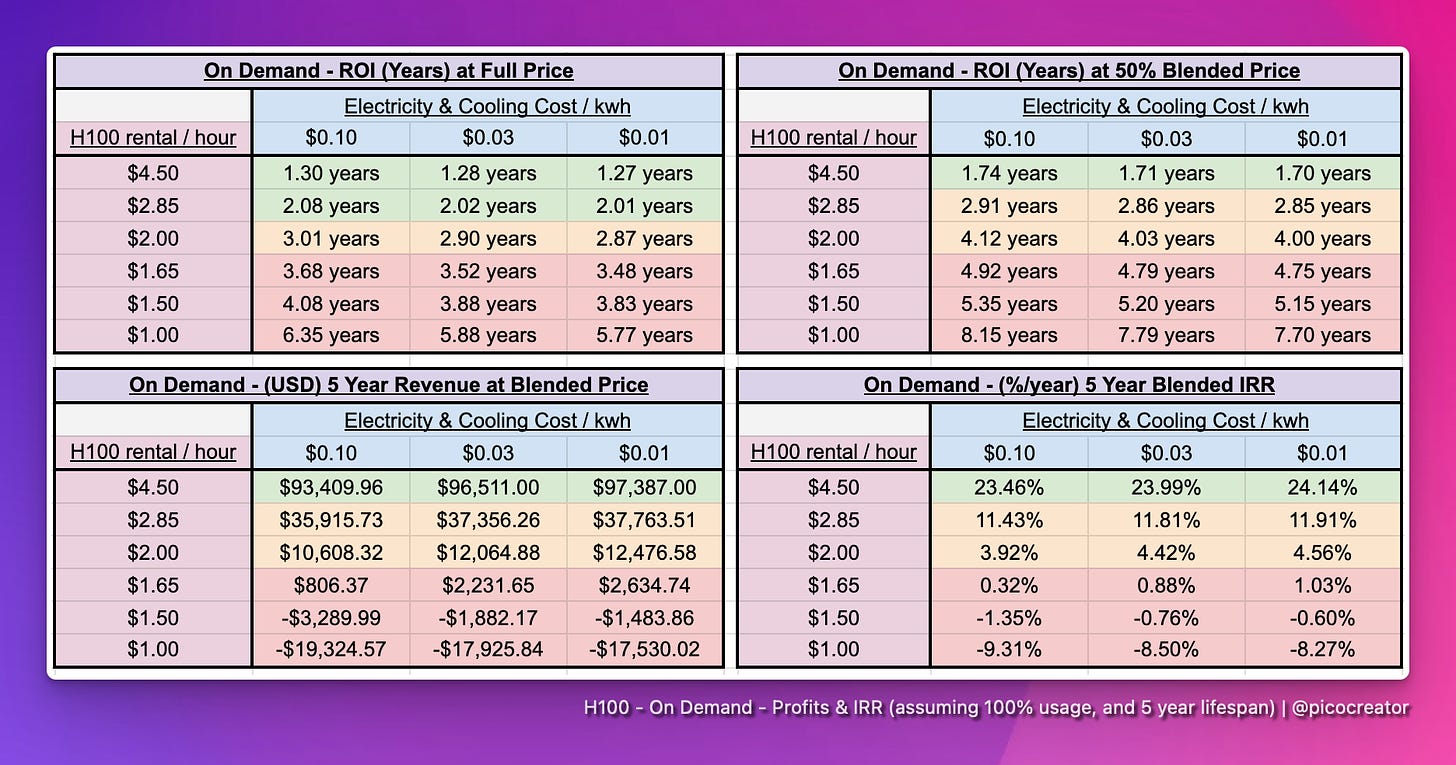
In summary, for an on-demand workload
>$2.85 : Beat stock market IRR
<$2.85 : Loses to stock market IRR
<$1.65 : Expect loss in investment
For the above ROI and revenue forecast projection, we introduced “blended price”, where we assume a gradual drop to 50% in the rental price across 5 years.
This is arguably a conservative/optimistic estimate given the >= 40% price drop per year we see now. But it’s a means of projecting an ROI while taking into account a certain % of price drop.
At $4.50/hour, even when blended, we get to see the original pitch for data center providers from NVIDIA, where they practically print money after 2 years. Giving an IRR (Internal rate of return) of 20+%.
However, at $2.85/hour, this is where it starts to be barely above 10% IRR.
Meaning, if you are buying a new H100 server today, and if the market price is less than $2.85/hour, you can barely beat the market, assuming 100% allocation (which is an unreasonable assumption). Anything, below that price, and you're better off with the stock market, instead of a H100 infrastructure company, as an investment.
And if the price falls below $1.65/hour, you are doomed to make losses on the H100 over the 5 years, as an infra provider. Especially, if you just bought the nodes and cluster this year.
Longterm reservation leases (3 years+)
Many infrastructure providers, especially the older ones - were not naive about this - Because they had been burnt firsthand by GPU massive rental price drops, after a major price pump, from the crypto days - they had seen this cycle before.
So for this cycle, last year, they pushed heavily for a 3-5 year upfront commitment and/or payment at the $4+ price range. (typically with 50% to 100% upfront). Today, they push the $2.85+ price range - locking in their profits.
This happened aggressively during the 2023 AI peak with various foundation model companies, especially in the image generation space, indirectly forced into high-priced 3-5 year contracts, just so to get to the front-of-the-line of a new cluster, and be first to make their target model, to help close the next round.
It may not be the most economical move, but it lets them move faster than the competition.
This, however, has led to some interesting market dynamics - if you are paying $3 or $4 per hour for your H100, for the next 3 years, locked into a contract.
When a model creator is done training a model, you have no more use for the cluster. What would they do? - they resell and start recouping some of the costs.
The current H100 value chain
From hardware to AI inference / finetune, it can be broadly viewed as the following
Hardware vendors partnered with Nvidia (one-time purchase cost)
Datacenter Infrastructure providers & partners (selling long-term reservations, on facility space and/or H100 nodes)
VC Funds, Large Companies, and Startups: that planned to build foundation models (or have already finished building their models)
Resellers of capacity: Runpod, SFCompute, Together.ai, Vast.ai, GPUlist.ai
Managed AI Inference / Finetune providers: who use a combination of the above
While any layer down the stack may be vertically integrated (skipping the infra players for example), the key drivers here are the “Resellers of unused capacity” and the rise of “good enough” open weights models like Llama 3, as they are all major influencing factors in the current H100 economical pressures.
The rise of open weights models, on-par with closed-source models.
Is resulting in a fundamental shift in the market
Market Trends: The rise of open-weights models
↑↑ Increased demand for AI inference & fine-tuning
Because many “open” models, lack proper “open source” licenses, but are being distributed freely, and used widely, even commercially. We will refer to them collectively as “open-weights” or “open” models instead here.
In general, with multiple open-weights models of various sizes being built, so has the growth in demand for inference and fine-tuning them. This is largely driven by two major events
The arrival of GPT4 class open models (eg. 405B LLaMA3, DeepSeek-v2)
The maturity and adoption of small (~8B) and medium (~70B) fine-tuned models
Today, for the vast majority of use cases, enterprises may need, there are already off-the-shelf open-weights models. Which might be a small step behind proprietary models in certain benchmarks.
Provides an advantage with the following
Flexibility: Domain / Task specific finetunes
Reliability: No more minor model updates, breaking use case (there is currently low community trust that model weights are not quietly changed without notification in public API endpoints, causing inexplicable regressions)
Security & Privacy: Assurance that their prompts and customer data are safe.
All of this leads to the current continuous growth and adoption of open models, with the growth in demand for inference and finetunes.
But it does cause another problem…
Compounded collapse of small & medium model creators
↓↓ Shrinking foundation model creator market (Small & Medium)
We used “model creators” to collectively refer to organization that create models from scratch. For fine-tuners, we refer to them as “model finetuners”
Many enterprises, and multiple small & medium foundation model creator startups - especially those who raised on the pitch of “smaller, specialized domain-specific models”, are groups who had no long-term plans / goals for training large foundation models from scratch ( >= 70B ).
For both groups, they both came to the realization that it is more economical and effective to fine-tune existing Open Weights models, instead of “training on their own”.
This ended up creating a triple whammy in reducing the demand for H100s!
Finetuning is significantly cheaper than training from scratch.
Because the demands for fine-tuning are significantly less in compute requirements (typically 4 nodes or less, usually a single node), compared to training from scratch (from 16 nodes, usually more, for 7B and up models).
This industry-wide switch essentially killed a large part of smaller cluster demands.
Scaling back on foundation model investment (at small/mid-tier)
In 2023, there was a huge wave of small and medium foundation models, within the text and image space.
Today, however, unless you are absolutely confident you can surpass llama3, or you are bringing something new to the table (eg. new architecture, 100x lower inference, 100+ languages, etc), there are ~no more foundation model cos being founded from scratch.
In general, the small & medium, open models created by the bigger players (Facebook, etc), make it hard for smaller players to justify training foundation models - unless they have a strong differentiator to do so (tech or data) - or have plans to scale to larger models.
And this has been reflected lately with investors as well, as there has been a sharp decline in new foundation model creators’ funding. With the vast majority of smaller groups having switched over to finetuning. (this sentiment is combined with the recent less than desired exits for multiple companies).
Presently today, there is approximately worldwide by my estimate:
<20 Large model creator teams (aka 70B++, may create small models as well)
<30 Small / Medium model creator teams (7B - 70B)
Collectively there are less than <50 teams worldwide who would be in the market for 16 nodes of H100s (or much more), at any point in time, to do foundation model training.
There are more than 50 clusters of H100 worldwide with more than 16 nodes.
Excess capacity from reserved nodes is coming online
For the cluster owners, especially the various foundation model startups and VCs, who made long reservations, in the initial “land grab” of the year 2023.
With the switch to finetuning, and the very long wait times of the H100’s
(it peaked at >= 6 months), it is very well possible that many of these groups had already made the upfront payment before they made the change, essentially making their prepaid hardware “obsolete on arrival”.Alternatively, those who had the hardware arrive on time, to train their first few models, had come to the same realization it would be better to fine-tune their next iteration of models. Instead of building on their own.
In both cases, they would have unused capacity, which comes online via “Compute Resellers” joining the market supply….
Other factors causing an increase in supply & reduced training demand
1) Large model creators goes off public cloud platform
Another major factor, is how all the major Model Creators, such as Facebook, X.AI, and arguably OpenAI (if you count them as part of Microsoft) are moving away from an existing public provider, and building their own billion-dollar clusters, removing the demand that the existing clusters depend on.
The move is happening mostly for the following reasons:
Existing ~1k node clusters (which costs >$50M to build), is no longer big enough for them, to train bigger models
At a billion-dollar scale, it is better for accounting to purchase assets (of servers, land, etc), which has booked value (part of company valuation and assets), instead of pure expenses leasing.
If you do not have the people (they do), you could straight up buy small datacenters companies, who have the expertise to build this for you.
With the demand gradually weaning away in stages. These clusters are coming online to the public cloud market instead.
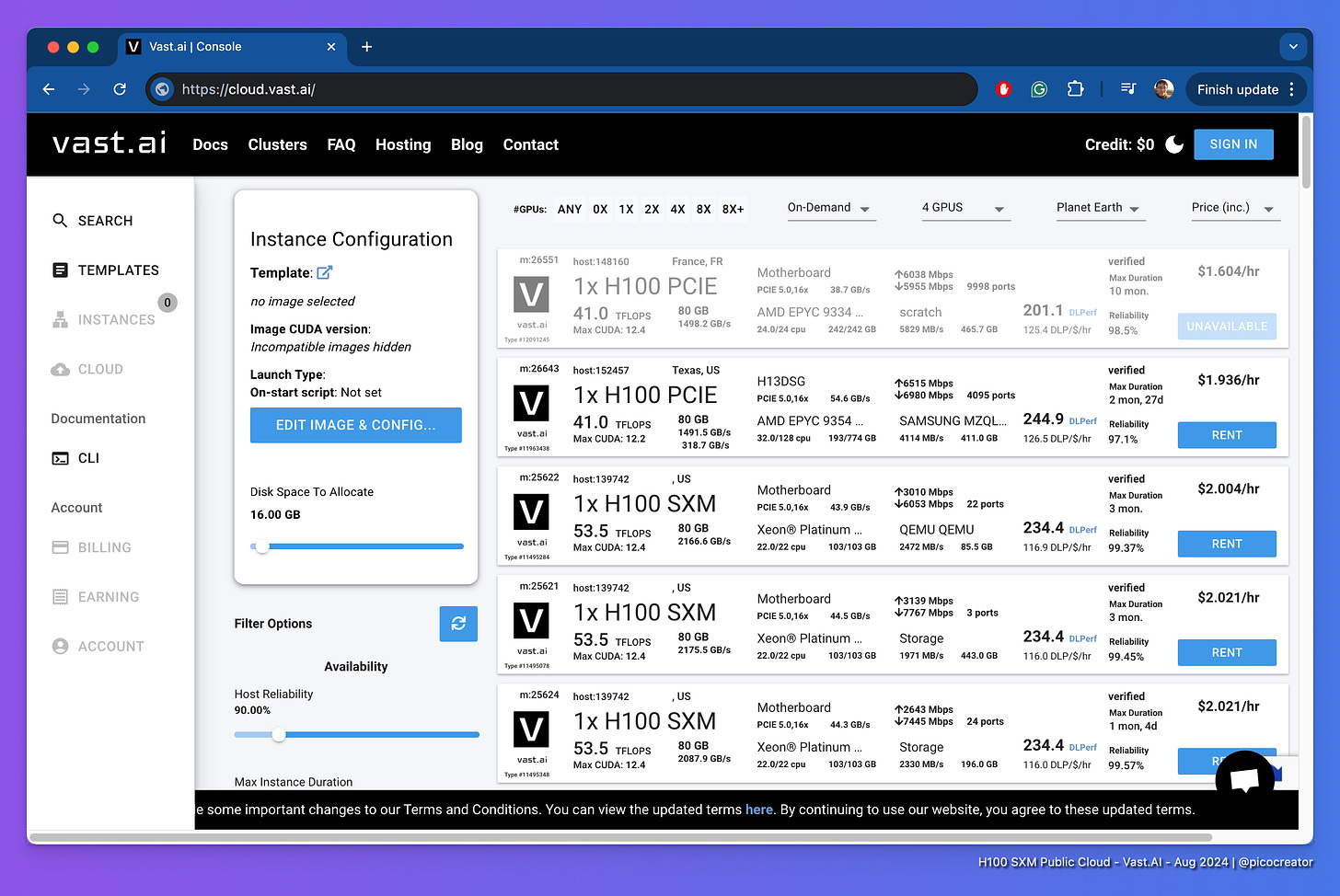
2) Unused / Delayed supply coming online
Recall all the H100 large shipment delays in 2023, or 6 months or more? They are coming online, now - along with the H200, B200, etc.
This is alongside, the various unused compute, coming online (from existing startups, enterprises or VCs as covered earlier).
The bulk of this is done via Compute Resellers, such as : together.ai, sfcompute, runpod, vast.ai, etc
In most cases, cluster owners have a small or medium cluster, (typically 8-64 nodes), that is underutilized. With the money already “spent” for the cluster.
With the primary goal is to recoup as much of the cost as possible, they rather undercut the market and guarantee an allocation, instead of competing with the main providers, and possibly have no allocation.
This is typically done either via a fixed rate, an auction system, or just a free market listing, etc. With the later 2 driving the market price downwards.
3) Cheaper GPU alternatives (esp. for inference)
Another major factor, is once your outside of the training / fine-tune space. The inference space is filled with alternatives, especially if your running smaller models.
One do not need to pay for the premium invoked by H100’s Infiniband and/or nvidia.
a) Nvidia market segmentation
H100 premium for training is priced into the hardware. For example nvidia themselves recommend the L40S, which is the more price competitive alternative for inference.
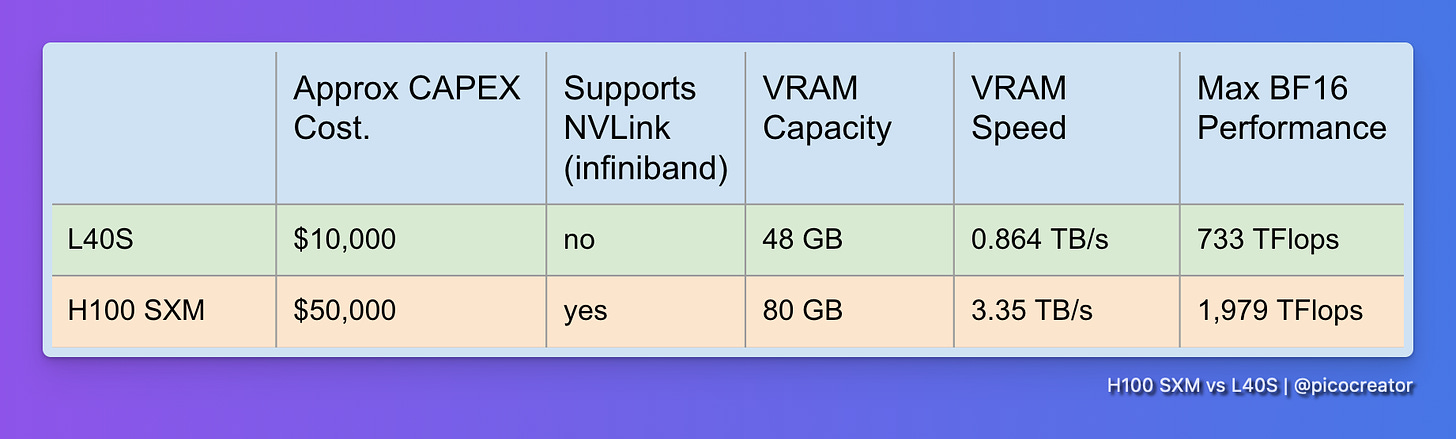
Which Is 1/3rd the performance, at 1/5th the price. But does not work well with multi-node training. Undercutting their very own H100 for this segment.
b) AMD and Intel alternative providers
Both AMD and Intel may be late into the game with their MX300, and Gaudi 3 respectively.
This has been tested and verified by us, having used these systems. They are generally:
Cheaper than a H100 in purchase cost
Have more memory and compute than a H100, and outperforms on a single node.
Overall, they are great hardware!
The catch? They have minor driver issues in training and are entirely unproven in large multi-node cluster training.
Which as we covered is largely irrelevant to the current landscape. To anyone but <50 teams. The market for H100 has been moving towards inference and single or small cluster fine-tuning.
All of which these GPUs have been proven to work at. For the use cases, the vast majority of the market is asking for.
These 2 competitors are full drop-in replacements. With working off-the-shelf inference code (eg. VLLM) or finetuning code for most common model architectures (primarily LLaMA3, followed by others).
So, if you have compatibility sorted out. Its highly recommended to have a look.
c) Decline of GPU usage in crypto/web3 space
With Ethereum moving towards proof of stake, ASIC dominating the bitcoin mining race, and the general crypto market condition.
GPU usage in mining for crypto has been a downward trend, and in several cases unprofitable. And has since been flooding the GPU public cloud market.
And while the vast majority of these GPUs are unusable for training, or even for inference, due to hardware constraints (low PCIe bandwidth, network, etc). The hardware has been flooding the market and has been repurposed for AI inference workloads.
In most cases if you are under <10B, you can get decent performance with these GPUs, out of the box, for really low prices.
If you optimize it further (though various tricks), you can even get large 405B models to run on a small cluster of this hardware, cheaper then an H100 node (which is what is typically used)
H100 Prices are becoming commodity-prices cheap.
Or even being rented at a loss - if so, what now?
What are the possible implications?
Neutral: Segmentation in H100 cluster prices
On a high level, it is expected that big clusters still get to charge a premium (>=$2.90 / hour) because there is no other option. For those who truly need it.
We are starting to see this trend for example with Voltage Park:
Where clusters with Infiniband are charged at a premium.
While the Ethernet-based instances, which are perfectly fine for inference are priced at a lower rate. Adjusting the prices for the respective use case/availability.
While there’s been a general decline in foundation model creator teams, it is hard to predict if there will be a resurgence, with the growth in open weights, and/or alternative architectures.
It is also, expected that in the future, we will see further segmentation by cluster sizes. Where a large 512-node cluster with Infiniband may be billed higher per GPU than a 16-node cluster.
Bad: New public cloud H100 clusters, late to the game, might be unprofitable - some investors may get burnt.
There is a lot against you, if you price it below $2.25, depending on your OPEX, you risk potentially being unprofitable.
If you price it too high >= $3, you might not be able to get sufficient buyers to fill capacity.
If you're late, you could not recoup the cost in the early $4/hour days.
Overall, these cluster investments will be rough for the key stakeholders and investors.
While I doubt it’s the case, if new clusters, make a large segment of the AI portfolio investments. We may see additional rippling effects in the funding ecosystem from burnt investors.
Neutral: Medium, to large Model creators, who purchased, long-term leases - already extracted value at the premium
Instead of a negative outlook, a neutral outlook would be some of the unused compute foundation model creators, coming online, are already paid for.
The funding market has already priced in and paid for this cluster and its model training. And “extracted its value” which they used for their current and next funding round.
Most of these purchases were made before the popularity of Compute Resellers, the cost was already priced in.
If anything, the current revenue they get from their excess H100 compute, and the lowered prices we get, are beneficial to both parties
If so the negative market impact is minimal, while overall it’s a net positive win for the ecosystem.
Good: Cheap H100s, could accelerate the open-weights AI adoption wave
Given that the open-weights model has entered the GPT-4 class arena. Falling H100 prices will be the multiplier unlock for open-weights AI adoption.
It will be more affordable, for hobbyists, AI developers, and engineers, to run, fine-tune, and tinker with these open models.
Especially if there is no major leap for GPT5++, because it will mean that the gap between open-weights and closed-source models will blur.
This is strongly needed, as the market is currently not sustainable. As there lacks the value capture on the application layer for paying users (which trickles down the platform, models, and infra layers)
In a way, if everyone is building shovels (including us), and applications with paying users are not being built (and collecting revenue and value).
But when AI inference and fine-tuning becomes cheaper than ever.
It can potentially kick off the AI application wave. If it has not already slowly started so.
Conclusion: Don’t buy brand new H100’s
Spending on new H100’s hardware is likely a loss-maker
Unless you have some combination of discounted H100s, discounted electricity, or a Sovereign AI angle where the location of your GPU is critical to your customers. Or you have billions and need a super large cluster.
If you're investing, consider investing elsewhere.
Or the stock market index itself for a better rate of returns. IMO
Featherless.AI plug …
What we do …
At Featherless.AI - We currently host the world’s largest collection of OpenSource AI models, instantly accessible, serverlessly, with unlimited requests from $10 a month, at a fixed price.
We have indexed and made over 2,000 models ready for inference today. This is 10x the catalog of openrouter.ai, the largest model provider aggregator, and is the world’s largest collection of Open Weights models available serverlessly for instant inference. Without the need for any expensive dedicated GPUs
And our platform makes this possible, as it’s able to dynamically hot-swap between models in seconds.
It’s designed to be easy to use, with full OpenAI API compatibility, so you can just plug our platform in as a replacement to your existing AI API for your AI agents. Running in the background
And we do all of this; As we believe that AI should be easily accessible to everyone, regardless of language or social status.
why we decided to be different, from other inference providers…
On the technical side of things, related to this article.
It is a challenge having PetaBytes’s worth of AI models, and growing, running 24/7 - while being hardware profitable (we are), because we needed to optimize every layer of our platform, down to how we choose the GPU hardware.
In an industry, where the typical inference provider pitch is typically along the lines of winning with their, special data center advantages, and CUDA optimization that they perform on their own hardware. Hardware is CAPEX intensive. (Which is being pitched and funded even today)
We were saying the opposite, which defied most investors’ sensibilities - we were saying we would be avoiding buying new hardware like the plague.
We came to a realization, that most investors, their analysts, and founders failed to realize, thanks to the billions in hardware investments to date. GPUs are commodity hardware. Faster than all of us expected.
Few investors have even realized we have reached commodity-level prices at $2.85 in certain places, let alone loss-making prices of a dollar. Because most providers (ignoring certain exceptions), only show their full prices after quotation or after login.
And that was the trigger, which got me to write this article.
While we do optimize our inference CUDA and kernels as well. On the hardware side; We’ve bet on hardware commoditizing and have focussed instead on the orchestration layer above.
So for us, this is a mix of sources from, AWS spot (preferred), to various data center grade providers (eg. Tensordock, Runpod) with security and networking compliances that meet our standards.
Leveraging them with our own proprietary model hot swapping, which boots new models up in under a second. Keeping our fleet of GPUs right-sized to our workload, while using a custom version of our RWKV foundation model as a low-cost speculative decoder. All of which allows us to take full advantage of this market trend, and future GPU price drops, as newer (and older) GPUs come online to replace the H100s. And scale aggressively.
PS: If you are looking at building the world's largest inference platform, and are aligned with our goals - to make AI accessible to everyone, regardless of language or status. Reach out to us at: hello@featherless.ai
Head over to Eugene’s Blog for more footnotes on xAI’s H100 cluster we cut from this piece.
Additional Sources:
GPU data: Tech Power Up Database. The A100 SXM had 624 bf16 TFlops, the H100 SXM was 1,979 bf16 TFlops
Microsoft & AWS allocated over $40 billion in AI infra alone: Wall Street Journal
Nvidia investor slides for Oct 2014: page 14 has the pitch for “data centers”
Semi Analysis: deepdive for H100 clusters, w/ 5 year lifespan approx for components
Spreadsheet for : new H100 ROI (Aug 2024)
Spreadsheet for: H100 Infiniband Cluster math (Aug 2024)
Unless you have some combination of discounted H100s, discounted electricity, or a Sovereign AI angle where the location of your GPU is critical to your customers, or you have billions and need a super large cluster for frontier model training
The cited “600 Billion Dollars “ is about Sequoia David Cahn article
Thanks, nice essay. Just a nit: your scenarios don't seem to reflect the full impact of electricity. An H100 takes nearly a kW of electricity, so because we're assuming full utilization, the $0.01/0.03/0.10 kWh charges can approximately be subtracted from the rental rate. In that case, looking at the $1/hr scenarios, I'd expect the 3-year revenue projections to differ by a few percent, not a small fraction of a percent.
Very strong work. I have numerous confirming datapoints about GPU supply,